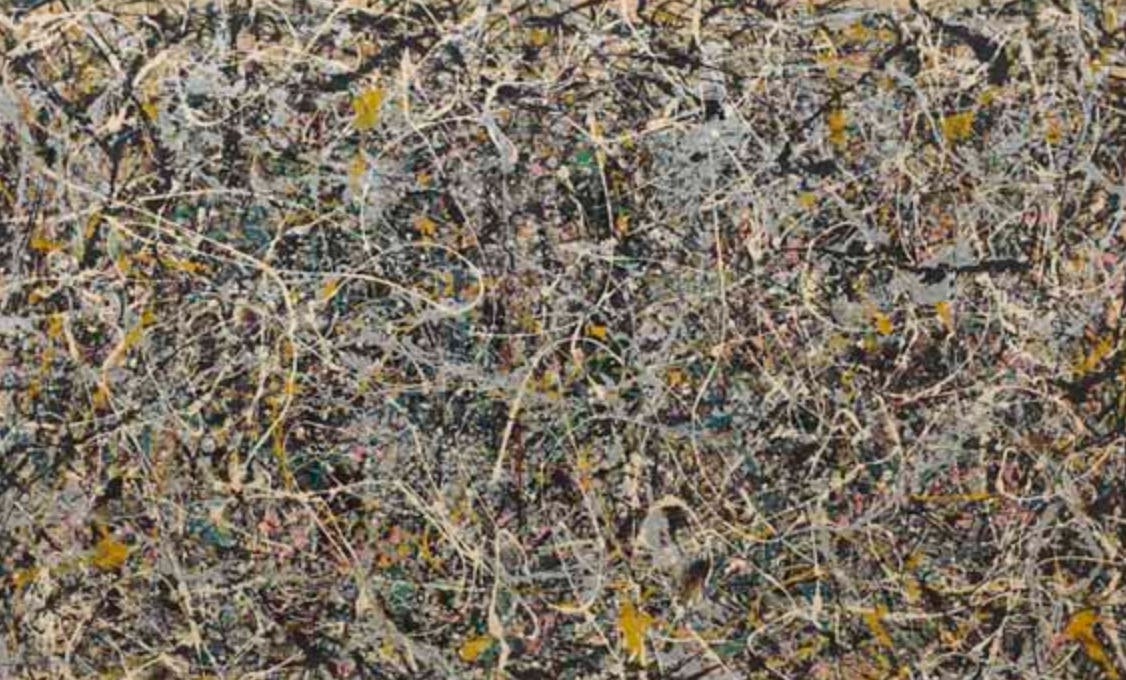
Art: Number 1, Jackson Pollock, 1949
There was a fantastic American game show in the 2000s, “Whose Line is it Anyway”, where the participants were given an improv scenario and had to act it out. The judges would then give them fake points for how well they did. The tagline of the show was, “Where everything’s made up and the points don’t matter,” The reason I think about it all the time is that, more and more I’m convinced that, in the Game of Life, there is no such thing as true, foundational data.
That’s crazy, you say. Vicki. You’re a data scientist, you say, and your job is to move datacloser to the truth so people can make decisions every day. Are you telling us that everything you’re doing is wrong?
Well, kind of. What I mean is that all the data that we trust and believe on a daily basis, is only accurate in a specific context, at a specific time, and at a specific level. If you dig deep enough, ultimately all of the data in the world that drives major and minor decisions alike is built on wobbly foundations.
Take, for example, the coronavirus mortality rate. We have no idea what the true number is. I mean we have some ideas of true numbers. But we’re not taking into account: undercounting minor cases that never get tested and never go to the hospital. Undercounting deaths that haven’t happened yet. Undercounting due to political reasons. Undercounting, simply because maybe hospitals are overwhelmed with the number of cases. Overcounting or undercounting recoveries. And much, much, more. As Elea says, human behavior is inconsistent and difficult to measure, and the virus is exactly an example of trying to measure human behavior on a large scale.
Randy wrote a great post about this idea,
As you can see, a LOT of people, are interested in denominators all of a sudden, because it matters a lot right now whether the big scary number that implicates your future mortality is an overestimate or underestimate.
A LOT of data science work is about working with denominators and coming to an understanding of what the denominator of a ratio means before drawing conclusions. People are getting a first-hand look at just how messy that process is, as they struggle with the frustration of wanting to know “the true number” and being utterly unable to get it because of measurement/definition issues. In their minds, the real death rate exists out in platonic space, but it’s inaccessible due to measurement.
Like Caitlin noted,
This isn’t the only place where there’s a lot of uncertainty about what the Truth as it exists in the world is. Where else are we bad at keeping track of stuff? Pretty much everywhere.
For example, in business.
Hidden Figures
I wrote a post a few years back about how most companies don’t really know how many users they have. Or rather, it’s not that they don’t know, it’s that the answer very highly depends on a number of circumstances.
Last week, Spotify created a list called “20 Million Thank Yous,” which it created when the service reached 75 million active users and 20 million premium subscribers.
This number is pretty impressive, but, as a data person, I was immediately curious how they came up with it, because there is no such thing as active users.
Well, there definitely is. There are people using Spotify all the time.
But what’s the definition of active?
Is it someone who’s signed up for Spotify?
Is it someone who’s signed up for Spotify and created a profile?
Is it someone who’s played one song?
Is it someone who’s played one song in the past minute? week? Month? Year?
Is it someone who spends 30 minutes on the site a day? What if they’re on the app but have the sound muted and forgot it’s playing?
Is it someone who likes to create playlists?
This problem is relevant across all industries, particularly those who operate on the web, and numerous companies have tried to tackle the issue in different ways.
No one has a single answer, because intrinsic human intellectual activity such as “listening,” “viewing,” or “using” is usually very hard to define.
This isn’t even digging into the issues around billing. In theory, if you pay for something, you’re a customer. But what if you’re on a free trial? What if your credit card expired and the company is trying to get you to verify it? What if we’re running the totals today but your membership ends tomorrow? What if you’re on some kind of special promotional plan?
There are other business numbers that are hard to pin down: user activity (I could do an entire post on how hard sessionization is.) How productive people are at work (I spent an entire internship working on a project around tracking white collar productivity and unsurprisingly never came up with an answer. BTW, this link is from 1988.) , and, believe it or not, revenue.
There is an entire debate around when and how to recognize revenue for any given company.
First, corporate financial statements necessarily depend on estimates and judgment calls that can be widely off the mark, even when made in good faith. Second, standard financial metrics intended to enable comparisons between companies may not be the most accurate way to judge the value of any particular company—this is especially the case for innovative firms in fast-moving economies—giving rise to unofficial measures that come with their own problems. Finally, managers and executives routinely encounter strong incentives to deliberately inject errors into financial statements.
Despite the raft of reforms, corporate accounting remains murky. Companies continue to find ways to game the system, while the emergence of online platforms, which has dramatically changed the competitive environment for all businesses, has cast into stark relief the shortcomings of traditional performance indicators
I personally have a hard enough time recognizing revenue for Normcore, and I’m a tiny newsletter. What if someone pays me $50 for a year’s subscription? Is it amortized over 12 months or should I recognize it for the month the day they pay me? What if someone cancels? What if someone adds halfway through the month? Imagine how hard it is for Fortune 500 companies with hundreds of lines of business, or LOBs as the consulting kids call them.
Ok, so the business world is built on vague handwavey-ness. What about economics? Economic numbers like GDP forecasts determine policy reactions from various politicians. GDP is the one number that’s absolutely solid, right?
Nope. If there’s anything that’s contested, it’s GDP, which does not include things like, and women’s domestic labor. Economists in other areas are not much better, particularly since they often use software like Excel to do their analyses, as a Nobel Prize winner did in 2014.
The spreadsheet was used to draw the conclusion of an influential 2010 economics paper: that public debt of more than 90% of GDP slows down growth. This conclusion was later cited by the International Monetary Fund and the UK Treasury to justify programmes of austerity that have arguably led to riots, poverty and lost jobs.
Now the mistake in the spreadsheet has been uncovered – and the researchers who wrote the paper, Carmen Reinhart and Kenneth Rogoff, have admitted it was wrong.
The correction is substantial: the paper said that countries with 90% debt ratios see their economies shrink by 0.1%. Instead, it should have found that they grow by 2.2% – less than those with lower debt ratios, but not a spiralling collapse.
They weren’t the only ones. Thomas Piketty, who wrote a hugely influential book on capital several years ago, had his numbers questioned, as well. These are just the public incidents we know about, by the way. There are probably hundreds of economic papers that talk about why GDP measurement is wrong and how to change it.
Ok, you say, well economics and business are handwavey by their very nature - they deal with human activity, which is nearly impossible to pin down at a large scale. We can’t expect them to get it right, anyway.
How about books? Books go out into the world, they’re read by millions of people. Surely books are correct? Well, apparently there’s not a lot of technical editing that goes into most of them.
In the past year alone, errors in books by several high-profile authors — including Naomi Wolf, the former New York Times executive editor Jill Abramson, the historian Jared Diamond, the behavioral scientist and “happiness expert” Paul Dolan and the journalist Michael Wolff — have ignited a debate over whether publishers should take more responsibility for the accuracy of their books.
Some authors are hiring independent fact checkers to review their books. A few nonfiction editors at major publishing companies have started including rigorous professional fact-checking in their suite of editorial services.
While in the fallout of each accuracy scandal everyone asks where the fact checkers are, there isn’t broad agreement on who should be paying for what is a time-consuming, labor-intensive process in the low-margin publishing industry.
Science-ish
How about science, you ask? Surely, science doesn’t change.
Surely scientific papers are vetted, rigid, produced by top researchers. We can be confident in science. Well…
In China, clinicians are expected to publish a certain number of research papers in international journals if they want to be promoted. The easiest way is to pay a paper mill, which seem to provide a full service: an English-speaking research paper containing Photoshop-generated fake research data, in a respectable peer-reviewed journal, with your name on it. Entire journals published by Wiley or Elsevier succumb to such scams, presumably because certain corrupt editorial board members are part of it. This was uncovered in an investigation byElisabeth Bik, as well as the pseudonymousSmut Clyde,Morty andTiger BB8, and narrated below by Smut Clyde.
The presently over 430 papers were traced to one specific paper mill not because of direct image reuse, but because the data showed same patterns of falsifications: same blot backgrounds, same shapes of bands, and similarly falsified flow cytometry plots.
Ok, sure, there might be some shady science journals.
But how about the basics of science?
Gravity? That’s changed, too.
And so has body temperature:
Despite what you were likely told in elementary school, the average human body temperature is not 98.6°F.
That commonly cited temperature dates to the 1850s, when a German doctor crunched the figure from data on 25,000 people in Leipzig. More recently, researchers found in a study of 35,000 British people that average body temperature is a bit lower, more like 97.88°F. That raised the question of whether average human body temperature might decrease over time.
I could go on, but I don’t want to cause pandemonium.
What does it all mean then?
The point is that, whatever data you dig into, at any given point in time, that looks solid on the surface, will be a complete mess underneath, plagued by undefined values, faulty studies, small sample problems, plagiarism, and all of the rest of the beautiful mess that is human life.
Just as all deep learning NLP models are really grad students reading phone books, if you dig deep enough, you’ll get to a place where your number is wrong or calculated differently than you’ve assumed.
George Box famously said, “All models are wrong, some are useful.” It might seem alarming, but in reality, it’s kind of reassuring, because it means we can stop trying to get to “the source of truth” and start looking at our data in a different light.
If no number is the complete truth, then there is no such thing as the real truth, and every single truth is just a relative version of the truth, much like Plato’s cave.
When we realize this, we can examine those relative versions and look at their own merits and flaws more critically. Instead of news sources trying to report a single mortality rate as the true rate, why not offer several rates and explain in detail how each is calculated? Instead of reporting on monthly average users, why not report on monthly average users, churn (users who leave the platform), users who are about to churn, and actives/inactives? Instead of GDP, why not report on productivity per sector? Why not report several different versions of GDP with different things factored in?
I realize I’m getting a little whimsical here: If we report five different numbers, people will never be able to understand what’s going on because we’re wired to make decisions very quickly. And if you’ve ever tried to present different courses of actions to stakeholders, you’ve probably been met with blank stares when you talk about things like confidence intervals, probabilities, and different scenarios. (Just projecting here, absolutely not a personal experience at all.)
But the reality is that the world we live in is extremely messy, depends on a lot of moving, different variables, and is always shifting under our feet. And the other thing to note, is that numbers only become solid after a long, long time.
So the more context-filled information we give and receive, the better it is.
And that, maybe, is the only real truth out there.
What I’m reading lately:
Learned this about PyPI recently:
Pypi, the Python package repository, costs 800k$/month in hosting: the volume that it serves brings real costs. Side note: our Continuous Integration infrastructures are really inefficient and contribute to this cost.@pwang @gjbernat @dave_hirschfeld @zooba @ncoghlan_dev @teoliphant @GaelVaroquaux @ChristianHeimes @pradyunsg @bitecode_dev @bigreddot @anacondainc Like we’re over 800k/month in hosting costs right now (some of that is due to one of our providers dropping their super cheap bandwidth tiers past a certain amount of bandwidth, and we’re working on reducing that, but still it’s expensive to operate)Donald Stufft @dstufftAll the different types of niche Twitter
Judd is out here doing all the important journalism at Olive Garden
Once again recommending Protocol newsletters for tech news if you don’t subscribe yet.
Simply shocked to find out AI startups are inefficient
This guy gave up $50k to write..this post
The Newsletter:
This newsletter’s M.O. is takes on tech news that are rooted in humanism, nuance, context, rationality, and a little fun. It goes out once a week to free subscribers, and once more to paid subscribers. If you like it, forward it to friends and tell them to subscribe!
The Author:
I’m a data scientist. Most of my free time is spent wrangling a preschooler and a baby, reading, and writing bad tweets. Find out more here or follow me on Twitter.
Discovering this post after you linked to it in light of the Twitter kerfuffle - so good! Lays out a lot of frustrations, some of which I was only mildly aware of, in a clear and funny way. Much appreciated!
I'm liking this kind of content. Just enough pessimism and optimism 👀